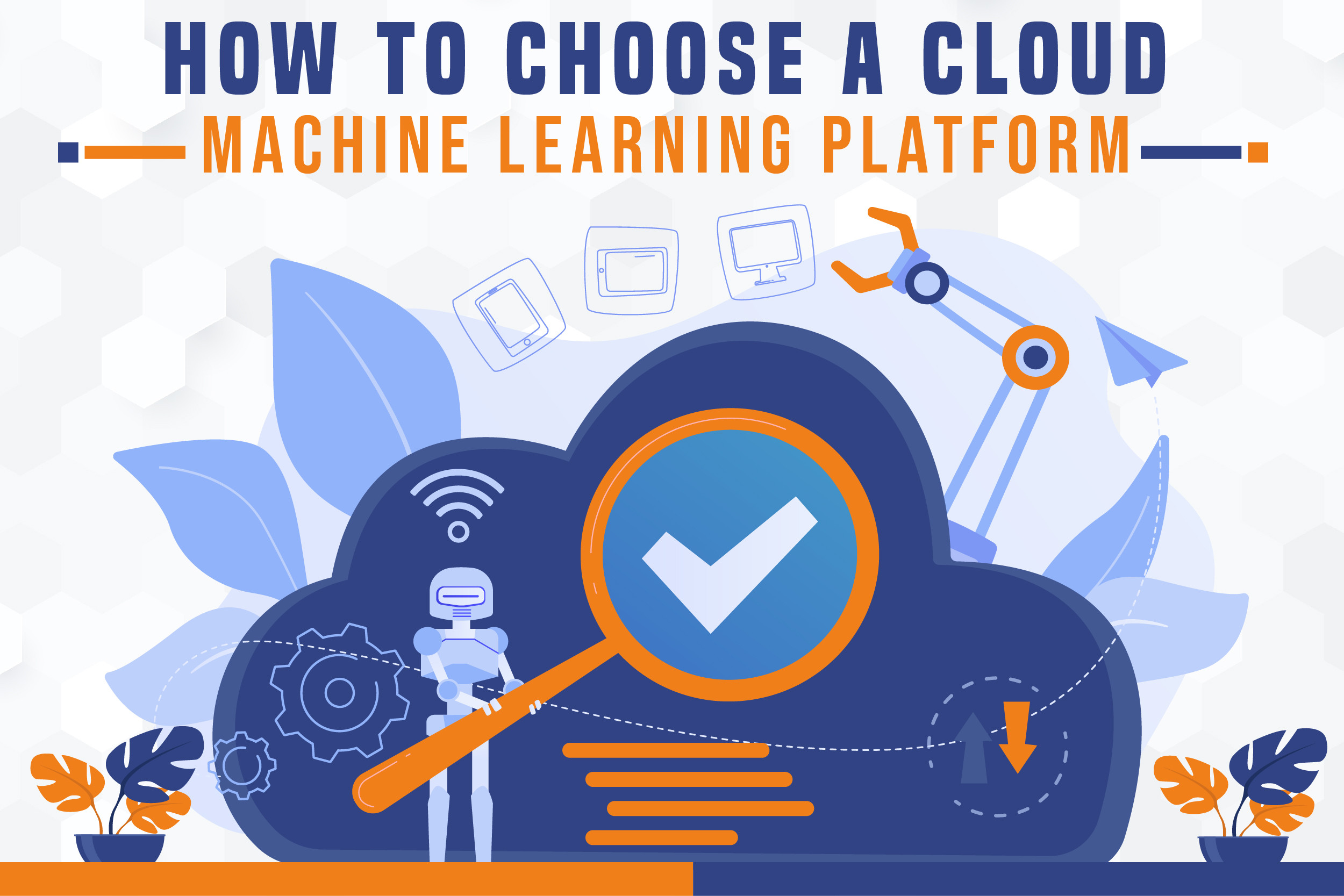
How To Choose A Cloud Machine Learning Platform?
Choosing a cloud machine learning platform can be a daunting task, especially with the numerous options available in the market today. It's important to understand the specific needs and goals of your organization, and how different platforms align with them. Whether it's cost efficiency, scalability, or ease of use, various factors need to be considered when making a decision. With so much at stake, it's crucial to thoroughly evaluate the options and determine the best fit for your organization.
The major cloud providers, as well as a few smaller clouds, have worked hard to develop their machine-learning platforms so that they can facilitate the whole machine-learning process, from project planning through model maintenance. Which of these clouds would best suit your requirements and how do you decide? Here are 12 features that every complete machine-learning platform needs to have.
Key Features To See For Choosing An Ideal Machine Learning Platform
1. You Must See If The Cloud Machine Learning Platform Is Close To Your Data
It is important to consider the proximity of the cloud machine learning platform to your data for several reasons:
- Latency:
The distance between the platform and your data can affect the speed of data transfer, resulting in latency. The closer the platform is to your data, the lower the latency, leading to faster processing times.
- Data privacy:
Having the platform close to your data reduces the risk of data privacy violations that could occur during transit.
- Cost efficiency:
Storing data in a distant location can result in increased costs, especially when it comes to bandwidth usage. Keeping the platform close to your data can help reduce these costs.
- Compliance:
Some industries have regulations that dictate the location of data storage. Having the platform close to your data ensures that you remain compliant with these regulations.
Considering these factors, it's important to verify the proximity of the cloud machine-learning platform to your data in order to ensure optimal performance, cost efficiency, and compliance.
2. Cloud Machine Learning Platform Must Support An ETL Or ELT Pipeline
A cloud machine learning platform must support an ETL (Extract, Transform, Load) or ELT (Extract, Load, Transform) pipeline to be effective in processing and utilizing large amounts of data. The pipeline is essential for preparing and cleaning data for analysis and modeling. In the ETL process, data is extracted from various sources, transformed into a usable format, and loaded into a data warehouse for analysis. In ELT, the data is first loaded into the data warehouse and then transformed.
The ability to support an ETL/ELT pipeline is crucial for any cloud machine learning platform, as the data processing step is often the bottleneck in the overall process of deploying machine learning models and generating insights. A platform that supports an efficient pipeline enables organizations to quickly and easily prepare and analyze data, leading to faster and more accurate results. Hence there is a dire need for extensive Technical Learning.
3. Must Be Compatible With Online Environment For Model Building
A cloud machine learning platform must be designed to work seamlessly with online environments to enable efficient model building. This means that it should be able to integrate with various data sources, tools, and infrastructure that are commonly used in the cloud.
The platform should also have robust collaboration features to allow data scientists, developers, and other stakeholders to work together in real-time. With compatibility with online environments, cloud machine learning platforms enable organizations to take advantage of the scalability, accessibility, and cost-effectiveness of the cloud to build and deploy AI models at scale and make the Technical Transformation effective.
4. Must Facilitate Scale-up and Scale-out Training
In addition to being compatible with online environments, a cloud machine learning platform must also support scale-up and scale-out training. Scale-up training involves using larger computing resources, such as more powerful GPUs, to train a single model. Scale-out training involves training multiple models in parallel using multiple GPUs, or even multiple cloud instances.
The ability to scale training resources is critical for organizations that need to handle large and complex datasets, or that need to train models quickly to meet business needs. A cloud machine learning platform that supports scale-up and scale-out training enable organizations to optimize their training process and reduce the time to market for their AI models.
5. Must Accommodate The Best Machine Learning And Deep Learning Frameworks
Also, most certainly it's crucial to check if the cloud machine learning platform is compatible with the best machine learning and deep learning frameworks. The most widely used machine learning and deep learning frameworks, such as TensorFlow, PyTorch, and sci-kit-learn, have proven to be effective for building and training AI models.
Supporting these frameworks allows organizations to leverage existing knowledge/ Technical Learning and experience and avoid vendor lock-in. Additionally, a cloud machine learning platform that supports multiple frameworks allows organizations to experiment with different approaches and find the best solution for their particular use case. The platform should also provide easy access to pre-trained models and pre-built components, as well as provide tools for tuning and deploying models, to further simplify the AI model-building process.
6. Must Provide Tuned AI Services
Your cloud machine-learning platform must be able to offer tuned AI services. These services provide pre-built and pre-configured AI models that can be easily integrated into applications, reducing the time and effort required to build AI models from scratch. The platform should offer a wide range of AI services that are optimized for different use cases, such as image classification, speech recognition, and natural language processing.
The platform should also provide easy-to-use APIs and pre-built integrations with popular development frameworks and programming languages, making it simple for developers to incorporate AI services into their applications. The use of tuned AI services can significantly accelerate the time to market for AI projects, enabling organizations to deliver value to their customers more quickly and effectively.
Wrapping Up
Hence, a cloud machine learning platform must have several key features to be effective and meet the needs of organizations looking to build and deploy AI models. The platform must be compatible with online environments, support scale-up and scale-out training, and offer support for the best machine learning and deep learning frameworks.
Additionally, the platform must provide tuned AI services that are pre-built and pre-configured, reducing the time and effort required to build AI models from scratch. Including(but not limited to) these features, a cloud machine learning platform provides organizations with the tools and infrastructure they need to build and deploy AI models at scale, enabling them to quickly and effectively deliver value to their customers.